One of the best ways to get inside the minds of your customers and understand their pain points is to gather their feedback through surveys. However, it’s important that you go about gathering this data in the right way so that you’re getting an accurate view of what your customers really think. Avoiding survey response bias is essential to extracting quality insights from customer feedback and getting accurate satisfaction metrics, insights that will actually resonate with your customer base.
If you don’t take the proper precautions in crafting your survey questions, you risk misunderstanding how your customers feel. The data will be inadequate and could lead to a wasted investment in initiatives that won’t close the feedback loop. Take a look at our breakdown on response bias below to learn more about what causes it and what easy checks you can take to avoid it in your surveys.
Response Bias Definition and Main Causes
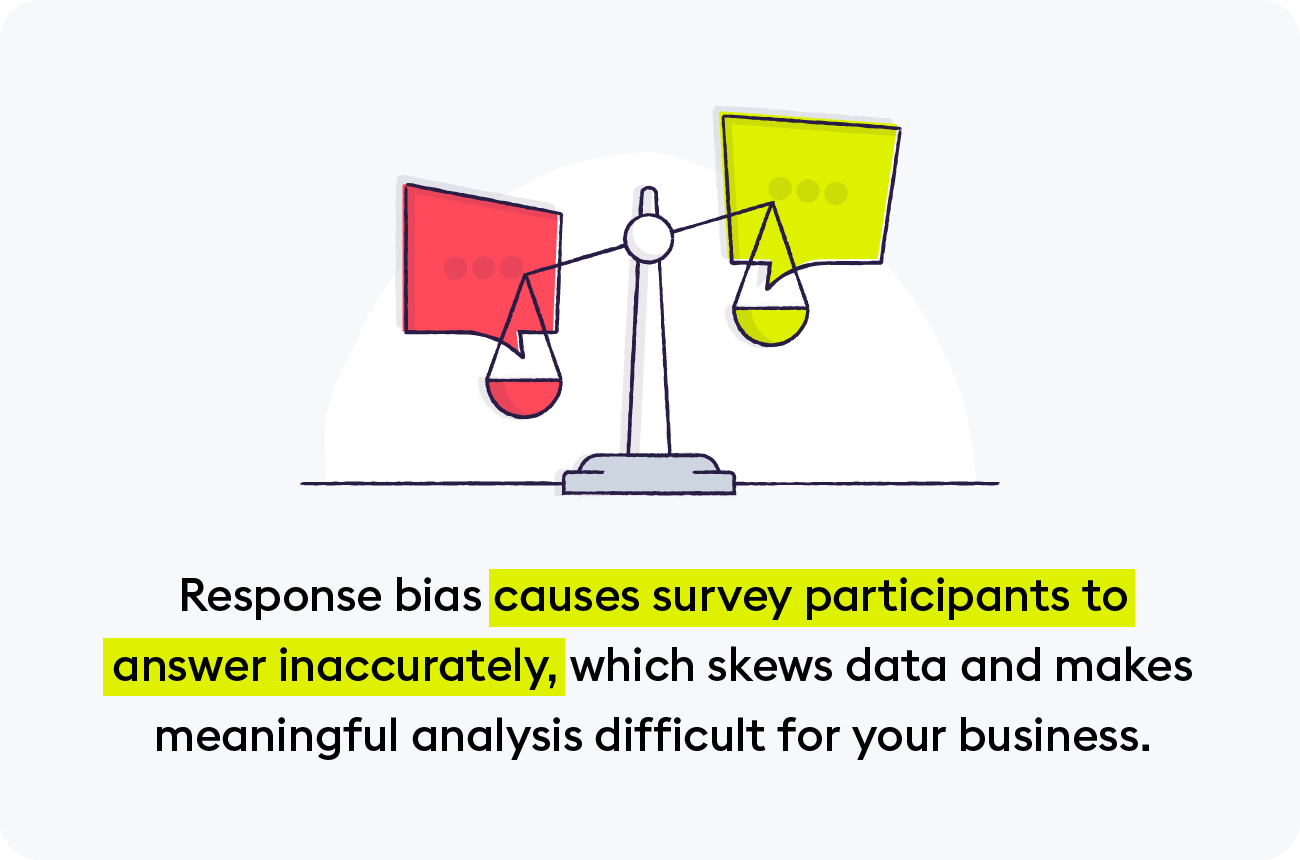
Response bias is anything that causes survey respondents to give inaccurate results. Respondents could answer questions inaccurately for a number of reasons; maybe they were confused about what the question was asking or wanted to portray themselves in a more flattering light through their answers.
Below are the five most common response bias causes along with examples for each:
1. Leading Questions, AKA Acquiescence Bias
Using leading questions is one of the most common ways to produce response bias, and it can be tricky to avoid when you are so steeped in the project. Leading questions are phrased in a way that subtly directs respondents to choose a particular answer, and this answer may not be the one that actually represents their feelings.
ExampleQ: “Would you say we did a good job with our new product rollout?”A: Yes or No
This question is subtly leading the respondent to acquiesce, or agree, with the implication that they are satisfied with the new product rollout. A better way to phrase this would be “How do you feel about our product rollout?” and provide several options to choose from.
2. Unflattering Results, AKA Social Desirability Bias
This type of response bias deals with the respondent’s internal perception of what the answers say about them as a person. People might not want to answer truthfully if their answer portrays them in an unflattering light.
ExampleQ: How many cigarettes do you smoke per day?A: 1-2, 3-4, 5-6, 7+
Respondents might incorrectly choose a lower number because they feel that’s a more socially-acceptable answer, and therefore they won’t be judged as harshly. In order to combat social desirability bias, make sure you stress to participants that the survey is completely anonymous. You may also want to keep the purpose of the survey under wraps so participants aren’t aware of how their responses will affect the findings.
3. All or Nothing, AKA Extreme Response Bias

“All or nothing” responding is when participants either mark all answers as “extremely agree” or “extremely disagree,” or mark all answers in the middle as “neutral”. This often happens when respondents get bored because a survey is too long. In order to combat this, make sure surveys take no longer than 5-10 minutes to complete and that participants are prepped on survey length beforehand.
You can avoid this issue by using new surveying techniques in AI as well. By analyzing survey data using AI, you don’t need to ask an exhaustive list of closed-ended questions to make sure you cover every theme you want feedback on. Instead, you can ask fewer open-ended questions and extract the different themes and insight at scale.
ExampleQ: How would you rate your experience with our mobile app? How do you feel about our customer service team? What has been your experience with our daily newsletter?A: Extremely satisfied, Extremely satisfied, Extremely satisfied
You should also make sure to go through your responses at the end and consider weeding out any that look like the participant chose the same answer every time to get through the survey as fast as possible.
4. Changing Context, AKA Question Order Bias
Question order bias is when participants are “primed” by the context of a previous question, which affects their answer to subsequent questions. Answering later questions incorrectly could be due to wanting to remain consistent across the survey, or because the first question made them think about the issue in a different way.
ExampleQ1: What’s the most important factor to you when voting for a political candidate?Q2: Do you think POLITICIAN is a worthy candidate for office?
If this person answered “the economy” for the first question, but the candidate in the second question doesn’t focus on the economy as one of their key issues, then the respondent will most likely view them negatively when answering the second question. However, if asked the second question first they might have viewed the politician more neutrally or even favorably.
In order to combat question order bias, it’s important to randomize the order in which questions are asked.
5. Overeager Participants, AKA Demand Characteristics
Demand characteristics are a broad area of psychological study, but in the context of survey response bias, it’s when your participants are too eager to help. This may sound like a good thing, but overeager participants tend to respond inaccurately because they want to help confirm the survey’s findings.
ExampleQ: How often do you play mobile games?A: Every day, Several times a week, Rarely, Never
Participants may consciously or unconsciously try to figure out what you’re studying in your survey research and respond accordingly. In the example above, say you are a mobile gaming company studying whether men use mobile games more than women. If a participant is able to figure out the purpose of the study, they may change their answer to confirm your theory. To avoid this, try to reveal as little as possible about the study and even the company if possible.
Other Types of Response Bias
We’ve covered the most common types of response bias in surveys, but there are a couple of other terms that savvy surveyors should know about:
Non-Response Bias

Non-response bias occurs when there is a significant difference between the people who responded to your survey and those that didn’t. Basically, it’s not a random sample of your customers anymore if the only ones that responded all have a common characteristic.
Example: You send out a direct mail survey to customers in a certain city.
Outcome: The majority of people who took the time to mail their responses were retirees, who presumably have more time than those of working age.
To avoid non-response bias, make sure surveys are short and easy to respond to (ie online questionnaires vs mail-in answers). It also helps to establish a relationship with customers you’d like to survey beforehand, as those with a connection to your brand are more likely to want to interact with you more. It’s also important that you have the ability to segment your customer data by behavior and demographics before you send the survey.
Voluntary Response Bias
Voluntary response bias can happen when you only include participants in your survey who specifically volunteer to answer your questions. Most of the time, these volunteers will already have some connection to the issue being discussed, and so won’t be able to answer the questions impartially.
Example: You want to gather data on a controversial topic like facial recognition software, so you email a college computer science department to see if students would like to volunteer for the survey.
Outcome: You’ll likely only get volunteer respondents with strong opinions one way or the other instead of results that represent the broader college student population.
To avoid voluntary response bias, it’s best to not rely on volunteer respondents whenever possible. You can also offer greater rewards or incentives for participating in order to appeal to a broader scope of people.
How to Structure Surveys to Avoid Response Bias
Now that you know what causes response bias in surveys, below are some actionable tips for making sure your study doesn’t fall prey. We’ve also included a checklist that you can take with you and use to QA future customer surveys.

1. Use Language Anyone Can Understand
Survey respondents won’t be able to give you accurate results if they can’t understand what you’re asking them. Make sure questions are simple and straightforward, with no hidden meanings or unnecessary descriptions. It’s best to use short, common words and phrases to make sure your questions are understood correctly.
Pro Tip: Run your questions through a content analyzer and make sure the reading level is no higher than a high school level.
2. Don’t Lead Respondents to a Specific Answer
As we discussed in the leading questions section, this is one of the most common ways to produce response bias in your surveys. To avoid this, make sure you are structuring your questions as openly as possible without any unnecessary adjectives. Put yourself in your customers’ shoes - would you feel comfortable replying “no,” or is the question pressuring you to say “yes?” Be sure to randomize answers as well to avoid priming.
Pro Tip: Utilizing questions such as “how do you feel about...” with a range of answers is typically more accurate than a “yes or no” question when it comes to opinion questions.
3. Consider the Knowledge Level of Your Audience
Along with making sure the language you use is easy to understand, you should also think about any background information needed. This all depends on the audience you are surveying - for example, college students studying business and entrepreneurship will have a better understanding of the startup landscape than the average customer who receives your survey in an email.
Pro Tip: Empathy is the key here. Imagine yourself in your audience’s place, with none of the background knowledge you have now. Would the questions you’re asking make sense with that level of information?
4. Provide Background Information if Necessary
To add on to the previous point, sometimes providing just a bit of context is all that is needed to make sure you avoid response bias. If the topic you’re researching requires some political, historical, or other contextual knowledge, including a paragraph or two of background information along with the survey question can be a good way to ensure that your audience is all on the same page.
Pro Tip: Though a couple of paragraphs is fine to provide, it’s important not to go overboard. Respondents aren’t going to want to read through a page of information before answering, and text that is too long will likely lead to people choosing incorrect responses to finish the survey more quickly.
5. Target the Correct Audience
If you’re looking to gather data about a specific type of customer, you’ll need to make sure that you’re tailoring your questions to that audience and ensuring that your survey is reaching them through the right channels. For example, if you’re gathering data about how users feel about your app’s onboarding instructions, you won’t want to send the survey to people who have been active users of the app for years as they likely won’t remember the onboarding from so long ago.
You’ll also want to avoid solely relying on volunteer participants in order to combat non-response and voluntary response bias.
Pro Tip: It can be helpful to build personas for your survey audiences to think about what makes them tick and where they can best be reached. If you’re looking to target older customers, for example, you won’t want to try to find them on Instagram.
6. Always Provide an Out
Sometimes one of your survey questions won’t apply to a specific respondent, so it’s best practice to provide an out as one of the answer choices. Otherwise, your results could be skewed as people have no choice but to choose a response that doesn’t actually apply to them. For example, if you’re a makeup brand surveying users on their favorite types of products, you should offer an “I don’t wear makeup” option along with the other choices.
Pro Tip: It’s important to watch for extreme response bias here, as you could get users choosing the “out” option for every question just to get through the survey faster.
7. Disregard Obvious Outliers
To go along with the Pro Tip above, make sure you’re weeding out any obvious outliers when you begin to analyze the data. Any respondents who choose the same extreme answer for every question or write in gibberish to open responses are generally safe to remove from your data set.
Pro Tip: If possible, you should also look at the time it took participants to complete the survey. If the average completion time was two minutes and 30 seconds, yet one person took only five seconds, that’s a good indication they rushed through without actually reading the questions.
8. Keep the Purpose Under Wraps
To avoid the influence of demand characteristics and social desirability, make sure to reveal as little about the purpose of the survey as possible. Keep questions vague and provide only the most necessary details.
Pro Tip: Consider using third-party surveying tools so that there is more of a distance between the researchers and the customers taking the survey.
9. Thoughtfully Structure Your Survey
A commonly overlooked form of response bias comes from surveys without a coherent structure, where surveys end up being a loose collection of "nice to knows". The manner in which a survey is structured (order of questions) can have a massive impact on the results gathers and the insights that follow. Therefore, one should always keep in mind whether the order of the questions make sense and to also give the respondent a good survey experience, which ensures the highest quality responses.
Take a look at the infographic below for a summary of these tips and definitions:

Collecting and analyzing survey data is an excellent start to improving customer retention and brand loyalty, and counteracting response bias allows you to make informed decisions about your business using accurate data.
Combining survey data with unstructured customer feedback free of bias is truly the way to achieve best in class analysis. It provides the foundation for customer insights and analysis. Try analysing a survey response now to get a taste of our AI insight engine.
Get started today free trial see how we can help you understand and address your customers’ unique challenges.
Sources: Sage Research Methods | ScienceDirect | VeryWellMind | Stat Trek | Versta Research